What Will the 2020s Hold? Assessment Trends, Past and Future
A 50-year veteran of psychometrics — the science of measuring mental abilities and processes — offers a brief history and insights into the future of testing.
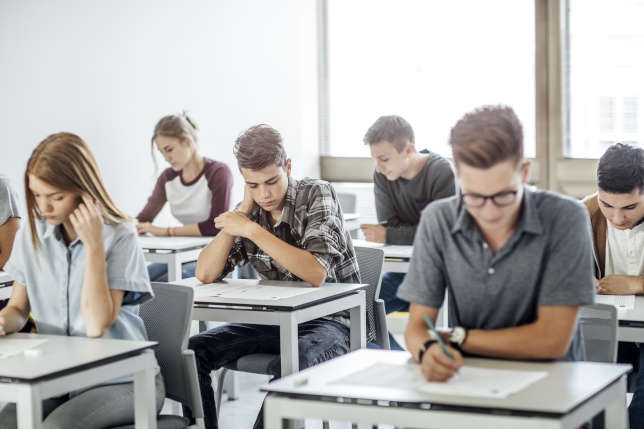
Adaptive testing has been around since at least the early part of the 20th century. The goal has always been to measure something — such as IQ, academic progress or personality traits — with the same precision as more traditional assessments but with fewer questions and less time, or with greater precision.
Early efforts had their drawbacks, of course, but we’ve come a long way in the intervening century or so. There is still much progress to be made, but some promising research today will likely change the way we assess students in the coming decade and beyond.
Early Adaptive Testing Efforts
An early example of adaptive testing was the Stanford-Binet intelligence test. Around since the early 20th century, it was administered differently from anything else at the time. Designed to assess examinees ranging in age from early childhood to adult, the Stanford-Binet consists of a battery of several distinct fixed-form subtests at each mental age level. The examiner uses available information about the examinee to start testing at an age level lower than the examinee’s expected mental age, then proceeds to administer subtests at different age levels until a basal age level and a ceiling age level are established. Subtests below the basal or above the ceiling level need not be administered, and the test score was based on performance within the levels that were used.
The adaptive nature of the test meant that one could reach a reasonably accurate score much faster than with a traditional test, but the necessity of a human administrator introduced human judgment and bias, which created errors in the measurement. Using computers to administer the test could eliminate that source of bias and error, but computers were not available when the first several versions of the test were developed.
Other researchers were interested in the area of adaptive testing, but Dr. David Weiss was one of the first to have some significant funding behind his research beginning in the early 1970s, when I joined him. Based at the University of Minnesota, Weiss was a counseling psychologist who explored the use of computers to administer IQ tests and assessments of personality traits and vocational interests.
When Weiss was in final negotiations with the Office of Naval Research on a contract to research computer-adaptive testing (CAT), I had just begun grad school at the university and was lucky enough to be invited to join the team. Weiss acquired what was called a “mini-computer” — it still took up most of a 9’ x 12’ room — that could drive four or five terminals for test-takers at a time. With 50,000 students at the university, we had a rich source of experimental subjects, and so we were off, trying to validate the concept of CAT and the many varied approaches to it.
Strengths and Weaknesses of CAT
The big advantage of CAT is that it can be done in about half the time that it takes to administer a comparably accurate measure of knowledge or skills with traditional assessments and without the bias of a human administrator in non-computerized adaptive tests. In an educational setting, of course, that means more time for curriculum and instruction. Adaptive testing has reduced testing time considerably: Tests that took three days before CAT now take one-and-a-half or two days.
Another advantage of CAT is that the scores are available immediately. There may be organizational delays, such as waiting for all students to take the test, but adaptive test scores can be available as soon as students are done.
A weakness of traditional CAT, or at least a common complaint about them, is that they almost exclusively comprise multiple-choice questions. Of course, that poses severe limitations in what can be asked. Critics say that adaptive tests don’t mirror how knowledge is put to use in the real world and thus don’t capture everything a student knows.
The alternative is a performance measurement in which one creates a realistic task, usually a complex one that involves numerous performance aspects, so that students can attempt to show what they know and their ability to apply that knowledge. This is far more complicated and time-consuming. Students take about 18 minutes on average to answer all 34 questions on the adaptive Star Reading assessment; the average Star Math assessment takes 22 minutes. In the same amount of time, a student might complete one or two performance measurement tasks, and there are likely several in a full assessment.
Those performance measurements yield rich information, but despite it being an issue of controversy for decades, the fact is that there is not a great deal of difference between performances measurement and CATs in terms of which students they identify as high, middle, and low achievers.
Better Data for Fairer — and More Accurate — Assessments
One promising trend in testing is less a new trend than the opportunity to pursue an old one, validity research, with better data. Those of us who have been involved in the research behind testing methods have lamented the difficulty of getting data on the kids who take our tests. That is different from the data that we collect in the course of taking the test itself — the results of the assessment — and is instead focused on who the test-taker is and whether the assessment may be unintentionally biased toward particular demographic sub-groups.
We are constantly either planning or conducting what we call validity research, where we develop and analyze evidence that tells us how well our tests are working as either predictors of how kids will do down the road or of estimating how they're currently doing on things that are important but that we can't test directly.
It is the critical role of any psychometrician to ensure that tests are fairly assessing various demographic subgroups. All test publishers use differential item functioning analysis to identify questions that may discriminate against one group or another, but this approach requires a lot of data. Comparing boys and girls is an obvious choice because the data set is large and easy to access. But, in the case of our Star Assessments, we may only receive data on which test takers are boys or girls about half of the time, and we may see an even lower response rate on information such as who is an English language learner or who has a disability, let alone what that disability might be.
It has historically been up to users to decide if they want to share that data with us. Most schools choose not to. In part, that’s because it doesn’t help them assess students, so they don’t see much use in entering it.
As schools see more and more utility (and security) in sharing data through secure systems with appropriate privacy protections in place, that data is becoming increasingly available. I’m optimistic that our recent acquisition of Schoolzilla will help us continue to refine our approach to assessments because the schools using it have already seen benefits integrating non-academic student data from Schoolzilla with academic data, and it’s already designed to separate the information we need from anything identifying the student personally.
Everyone’s trying to crack this nut, so I’m looking forward to seeing the progress we’ll make on it at Renaissance and in the field more broadly.
The Changing Use and Administration of Assessments
Schools typically test kids at the beginning of the year to screen who's high, who's low, and who ought to get special treatment, and then at the end of the year to determine who learned and who didn't. More frequent but less time-consuming assessment throughout the year can help guide differentiation and instruction. In cases that require frequent progress-monitoring, our tests can be used monthly or even as often as weekly, although three or four assessments in the course of the year should be enough to help teachers make decisions about individual students’ instruction. I think that the trend toward using assessments to guide instruction will accelerate in the coming years.
Kids will be grouped. Students will be treated similarly within their group, but differently across groups in an effort to bring everyone to the same point of competency. It probably won't fully succeed in bringing every student to the point we’d like to see them reach — it has never worked before in all the ways that have been tried — but I think it's a much more promising approach that we should pursue vigorously in the near future.
Another approach that I think we’re going to see more of in the long term is embedded assessments. These are tests that are folded into instruction so as to be indistinguishable. In theory, students won’t even know they’re being assessed, and the results should be available to inform instruction almost immediately.
This concept is new enough that it needs to be validated more. There will be some surprises (both happy and disappointing) as it’s developed and refined, but we’re likely to see a great deal of evolution on embedded assessments.
The Future of Assessment Tech
Increasingly, artificial intelligence (AI) applications are in development or use in educational settings, whether for assessment or instruction or a combination of the two. As with embedded assessments, the field still has a lot of shaking out to do, but as a whole it’s promising.
Again, I am optimistic while maintaining a healthy skepticism about changes like this. When new technologies come along, they need to be explored, just as computer-adaptive testing was explored. CAT succeeded. A lot of other technologies have fallen by the wayside for one reason or another, and we'll see that happen again with other possibilities AI will open for us.
We need to be mindful that even the most exciting innovations might fall short for one reason or another — without discouraging the younger researchers exploring these new avenues. They need resources and encouragement to explore the possibilities that unlock new methodologies and technologies that will improve assessment (and the instruction and learning it informs) as much as CAT has.